IMO 6: Resistance
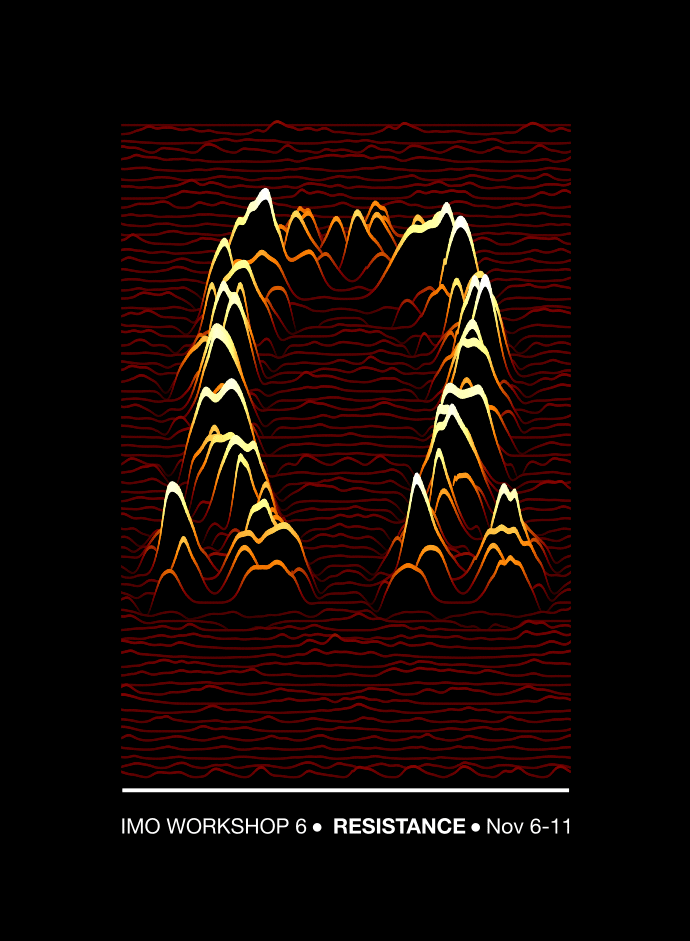
Resistance in Cancer
Drug resistance is currently the single biggest issue in cancer today. Despite the development of a new generation of molecularly targeted drugs, which showed initial promise, resistance remains the key driver of treatment failure. This is largely due to the heterogeneous nature of cancer, this heterogeneity bridges many scales: genetic, epigenetic, phenotypic, microenvironmental and seen both within (itra-tumour) and across patients. Developing treatments that do not ignore this heterogeneity and instead exploit it, has become part of an emerging paradigm of evolutionary enlightened therapies. However, treating cancer like a chronic disease means that we need a better understanding of the disease kinetics (both with and without drug). Mathematical models are an ideal tool for understanding how heterogenous cancers respond to therapy and can suggest smart strategies to maximize response whilst minimizing both resistance and toxicity. This workshop will consider all aspects of drug resistance through a range of cancers and treatments.
Presenting the Research Question
Team Orange
Clinician Eric Padron presents #TeamOrange question on myeloid neoplasms #MoffittIMO pic.twitter.com/SkkdvEKhD0
— Sandy Anderson (@ara_anderson) November 7, 2016
Team Red
Joel Brown defines the #TeamRed question on Triple Negative Breast Cancer #MoffittIMO pic.twitter.com/AWKTwLTVeO
— Sandy Anderson (@ara_anderson) November 7, 2016
Team Yellow
Sara Nizzero @snizzeros presents #TeamYellow question on Lung Cancer adaptive radiotherapy #MoffittIMO pic.twitter.com/SWyFhUXt04
— Sandy Anderson (@ara_anderson) November 7, 2016
Team Green
Chandler Gatenbee @cgatenbee explains #TeamGreen strategy in dealing with Melanoma treatment response & resistance #MoffittIMO pic.twitter.com/CwMsvcW0ma
— Sandy Anderson (@ara_anderson) November 7, 2016
Team Blue
Jill Gallaher @jillagal presents #TeamBlue question on Non-small-cell Lung Cancer #MoffittIMO pic.twitter.com/tX58QsHBYb
— Sandy Anderson (@ara_anderson) November 7, 2016
And the winner is...
Winning team: specific aims
"Dark selection for JAK/STAT-inhibitor resistance in CMML"
Chronic Myelomonocytic Leukemia (CMML) is an aggressive clonal myeloid disorder with a median survival of 34 months. Despite understanding the genetic makeup of the disease, adequate therapeutic options to eliminate, or at least control, the disease are still lacking. Recently, the JAK1/2 inhibitor ruxolitinib had been FDA approved for the related disease myelofibrosis, and shown promise in clinical trials for CMML. Even though ruxolitinib does not impact the load of leukemic progenitors in the bone marrow, the therapy substantially alleviates symptoms in ~80% of patients by reducing the load of inflammatory cytokines and accumulation of abnormal myeloid cells in the periphery. This improvement represents the best currently available therapeutic option for many patients. Unfortunately, this response does not last as both subjective and objective symptoms return after several months on therapy. The molecular mechanism of ruxolitinib resistance is thought to be due to the stochastic acquisition of heterodimers at cytokine receptors. However, the emergence of resistance to ruxolitinib represents an apparent evolutionary paradox. In contrast to other cases of resistance to targeted and cytotoxic therapies, ruxolitinib treatment does not reduce the population size of leukemic progenitor/stem cells, constituting units of Darwinian selection. Moreover, development of resistance is not associated with measurable changes in sub-clonal architecture of leukemias, further arguing against classical Darwinian selection for resistant heritable phenotypes. Before clinical strategies to overcome resistance can be developed, we must understand the underlying biological mechanisms that drive it. To this end we will employ the following specific aims.
Hypothesis
We hypothesize that understanding the mechanisms of selection will help to define strategies to overcome the emergence of resistance.
Specific Aim 1
To determine whether the emergence of ruxolitinib resistance is a Darwinian, Lamarckian, or non-cell autonomous process.
Objectives
- Characterize dynamic regimes of the 3 above processes using a common framework of stochastic processes.
- Identify experimental measurements and design relevant statistical tests to discriminate between the three models based on their characterization using approximate Bayesian computation.
- Experimentally determine proliferation rates and characterize changes in phenotypic architecture of human bone marrow CMML progenitors through the course of ruxolitinib therapy, using single cell technology.
- Gather time-series data of heterodimerization at cytokine receptors in a mouse model to distinguish which of the three process is consistent with data based on our characterization of their dynamics and developed Bayesian inferential pipeline.
Specific Aim 2
To determine the kinetics of cytokine expression and resultant symptomatology in patients treated with ruxolitinib.
Objectives
- Integrate the phenomenological model from specific aim 1 with microenvironmental parameters such as hypoxia and various mathematical models of cellular and cytokine dynamics within the bone marrow compartment using a hybrid discrete and continuous cellular automata multi-scale approach.
- Gather time series data of cytokine levels and symptomatology in patients during ruxolitinib therapy to parametrize the above mechanistic model.
Impact
This proposal will solve the paradox of acquired ruxolitinib resistance in the absence of detectable selective pressure. Our findings will inform the road to preventing/delaying ruxolitinib resistance in CMML. Furthermore, insights gained in this study will be applicable to a wider range of cancers whose therapy does not augment tumor burden.
Watch Scientists Inc's Video Summary
IMO Workshop 2016 from Scientists Inc on Vimeo.