IMO 3: Personalized Medicine
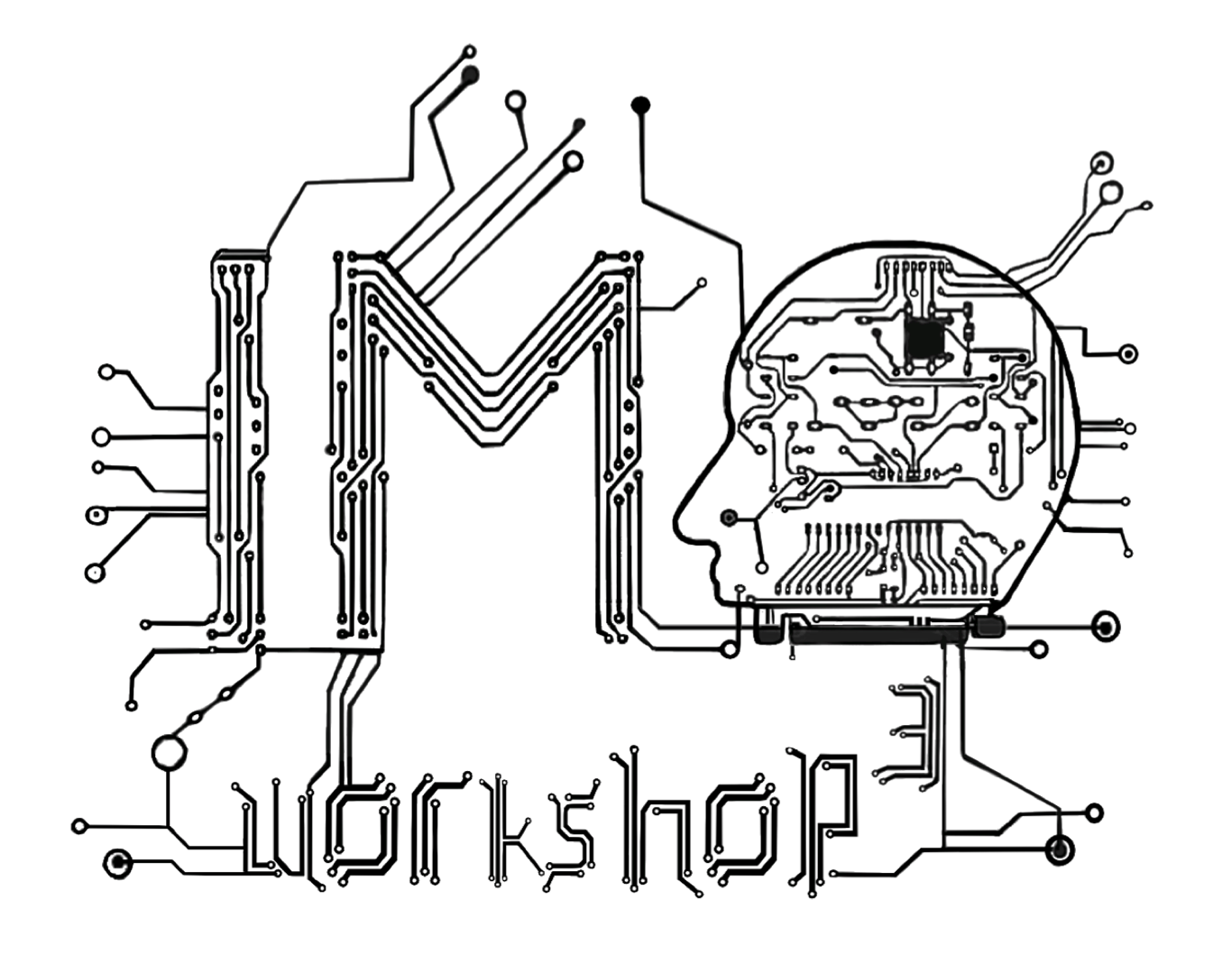
Personalized Medicine
Increasingly large, multiscale, and diverse data sets are being collected from patients with cancer. The most well-recognized sources of these data are high throughput molecular technologies, clinical imaging modalities and tumor biopsy analyses. While cancer may generate big data, big data are not cancer. Indeed, these big data sets have had limited impact on actual clinical practice, in part, because current standard of care treatment decisions are often made using guidelines that are statistically driven and based on averaged data from large patient cohorts with limited patient-specific inputs. Most cancers have at least a few effective therapies, but even these few produce a huge therapeutic parameter space of drug combination and dose schedules. Thus, an obvious challenge is developing models that exploit patient data sets to make better patient specific therapeutic decisions. This will be the central theme of our workshop this year.
And the winner is...
Winning team: specific aims
"A real-time adaptive treatment platform to extend survival in lung cancers with epidermal-growth factor (EGFR) mutation."
Significance
Non-small cell lung cancer is the leading cause of cancer-related mortality, with over 200,000 cases and 140,940 deaths estimated in the United States in 2011. This cancer is defined at the molecular level by driver mutations that occur in multiple oncogenes. Driver mutations begin in a single cell, and cause constitutive activation of signaling, leading to proliferation and tumorigenesis. Approximately 15-20% of tumors are characterized by activating mutation of the epidermal-growth factor receptor (EGFR). Although targeted therapy with oral tyrosine kinase inhibitors (TKIs) induces substantial tumor regression in the majority of patients, these responses are rarely durable, with median survival less than two years. This inevitable failure is due to emergence of diverse tumor resistance mechanisms that abrogate TKI activity. For example, selective pressure by EGFR TKI leads to a resistant clone harboring a gatekeeper mutation (T790M) in up to half of cases. Alternate acquired resistance mechanisms include overexpression of MET, human epidermal growth factor receptor 2 (HER2), and downstream mutation in phosphotidyl inosityl-3 kinase (PI3K). These clones often compete for survival within a dynamic fitness landscape inside the tumor microenvironment. Critical factors that impact clonal penetrance include choice of therapy and genetic hetereogeneity. Rationale selection of targeted agents, alone or in combination, may inhibit the emergence of multi-drug resistant clones, thus extending patient survival. The evolutionary complexity of these signaling pathways requires a quantitative approach which may be addressed using a patient-specific mathematical model.
Preliminary model
Signaling networks and clinical patient covariates were used to construct a mathematical model that predicts optimal drug sequence to maximize survival time and avoid escalating toxicity. Based upon systematic literature review, we utilized inputs such as published radiographic response rates, preclinical activity, and probability of mutation. We then applied increasingly sophisticated modeling techniques to inform future optimal courses of treatment. The inputs into the models are split into three categories, which also divide into the layers of complexity of the models used. The three types of input include: inferred constants throughout all populations, response parameters from each patient visit, and parameters from published patient-level case studies.
Hypothesis
Pretreatment molecular phenotype, in combination with real-time clinical variables obtained from plasma and radiographic imaging, can be used to predict optimal drug sequence and thereby extend progression-free survival. In the future, this adaptive mathematical model could guide clinical decision making for personalized therapy of EGFR-positive stage IV NSCLC.
Specific Aim 1
Refine the mathematical model, using clinical data from a retrospective institutional cohort of advanced EGFR+ lung cancer patients. We have identified five distinct targeted treatment groups (erlotinib, afatinib, BKM120, selumetinib, and onartuzumab + erlotinib) with linked tumor molecular profiles and clinical response data. Model inputs will include: presence of T790M, MET and HER2 amplification, PI3K mutation, estimated progression-free survival, and circulating hepatocyte growth factor (HGF) levels; as well as radiomics from computed tomography. The output of the model will be volumetric tumor burden. By performing parameter re-estimation on these data, a more refined clinical prediction tool can be derived. This model can then be tested in future prospective studies.
Specific Aim 2
Utilize model-derived novel drug combination schedules and compare with standard of care using a metastatic EGFR driven in vivo model. Using the mathematical model, we will identify treatment schedules that significantly reduce tumor burden and prolong survival in a virtual murine population. These putative treatment regimens, consisting of time-dependent single and/or combinations of targeted inhibitors, will be tested against erlotinib monotherapy in this mouse model of EGFR-driven tumors. Translational endpoints will include survival time, toxicity (animal weight), circulating HGF levels, and genotype of emerging resistant disease.
Watch Scientists Inc's Video Summary
IMO workshop 2013 from Scientists Inc on Vimeo.